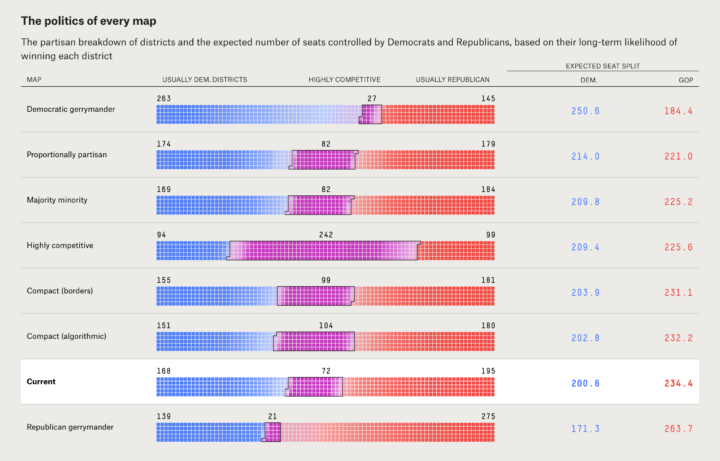
FiveThirtyEight asks, “There’s a lot of complaining about gerrymandering, but what should districts look like?” Looking for an answer, they imagined redistricting with different goals in mind, such as gerrymandering favoring Republicans or Democrats, promoting competitive elections, and maximizing majority-minority.
Check out the possibilities for the nation or zoom in to a specific state. The latter provides a further breakdown by district and then race. So yeah, if you’re into this stuff, set aside some time to poke at this one.